Abstract
Estimating eye-gaze from images alone is a challenging task,in large parts due to un-observable person-specific factors. Achieving high accuracy typically requires labeled data from test users which may not be attainable in real applications. We observe that there exists a strong relationship between what users are looking at and the appearance of the user’s eyes. In response to this understanding, we propose a novel dataset and accompanying method which aims to explicitly learn these semantic and temporal relationships. Our video dataset consists of time-synchronized screen recordings, user-facing camera views, and eye gaze data, which allows for new benchmarks in temporal gaze tracking as well as label-free refinement of gaze. Importantly, we demonstrate that the fusion of information from visual stimuli as well as eye images can lead towards achieving performance similar to literature-reported figures acquired through supervised personalization. Our final method yields significant performance improvements on our proposed EVE dataset, with up to 28% improvement in Point-of-Gaze estimates (resulting in 2.49 degrees in angular error), paving the path towards high-accuracy screen-based eye tracking purely from webcam sensors.
Preview Video
Acknowledgments
We thank the participants of our dataset for their contributions, our reviewers for helping us improve the paper, and Jan Wezel for helping with the hardware setup. This project has received funding from the European Research Council (ERC) under the European Union’s Horizon 2020 research and innovation programme grant agreement No. StG-2016-717054.
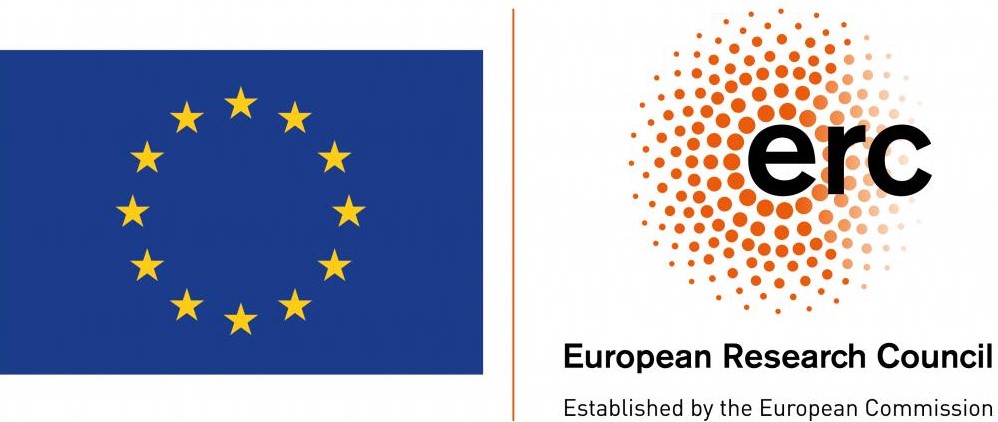
License
This Dataset is released under the CC BY-NC-SA 4.0 license with additional conditions and terms.
Please refer to the complete license file here.
Downloads
- The EVE Dataset is available on request. Please fill in this Google Form to gain access to the dataset.
- Public Leaderboard. Detailed instructions on how to produce predictions for the leaderboard is provided on our Codalab page. Paper authors should use this platform for reporting performance on the public portion of the EVE test set.