Many computer vision tasks can benefit from the disentanglement of factors. While some of the factors are task-relevant and labeled (e.g. gaze direction), others are extraneous and unknown a priori (e.g. lighting condition). We propose the Self-Transforming Encoder-Decoder (ST-ED), which learns to discover and disentangle extraneous factors in a self-supervised manner. We show that explicitly disentangling extraneous factors results in more accurate modelling of task-relevant factors. Furthermore, given limited amounts of labeled training data, our method allows for improvements in downstream tasks via data augmentation. Please check our overview video for the general approach.
Given an input image, our method can separetely control the gaze direction and head orientation with high-fidelity. Please check our supplementary video for more qualitative comparisons.
Overview Video
Supplementary Video
Acknowledgments
This project has received funding from the European Research Council (ERC) under the European Union’s Horizon 2020 research and innovation programme grant agreement No. StG-2016-717054.
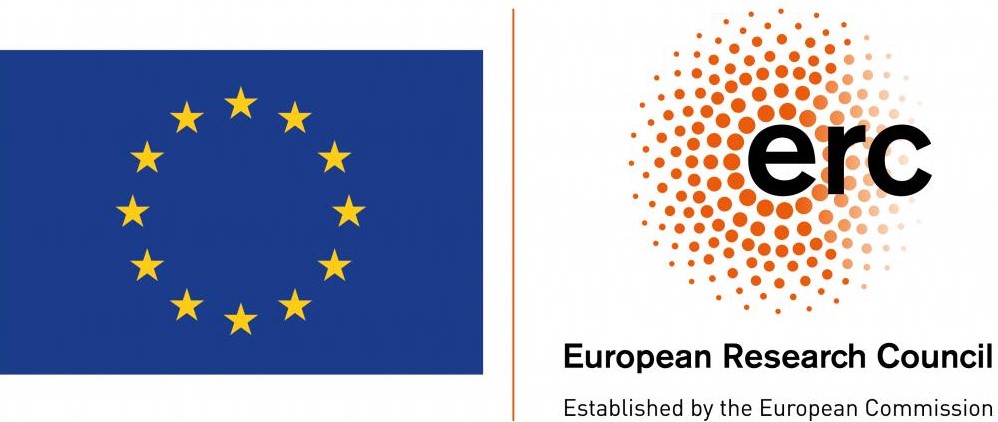